Created by me.
Link : https://huggingface.co/codeShare/JupyterNotebooks/blob/main/sd_token_similarity_calculator.ipynb
How does this work?
Similiar vectors = similiar output in the SD 1.5 / SDXL / FLUX model
CLIP converts the prompt text to vectors (“tensors”) , with float32 values usually ranging from -1 to 1.
Dimensions are [ 1x768 ] tensors for SD 1.5 , and a [ 1x768 , 1x1024 ] tensor for SDXL and FLUX.
The SD models and FLUX converts these vectors to an image.
This notebook takes an input string , tokenizes it and matches the first token against the 49407 token vectors in the vocab.json : https://huggingface.co/black-forest-labs/FLUX.1-dev/tree/main/tokenizer
It finds the “most similiar tokens” in the list. Similarity is the theta angle between the token vectors.
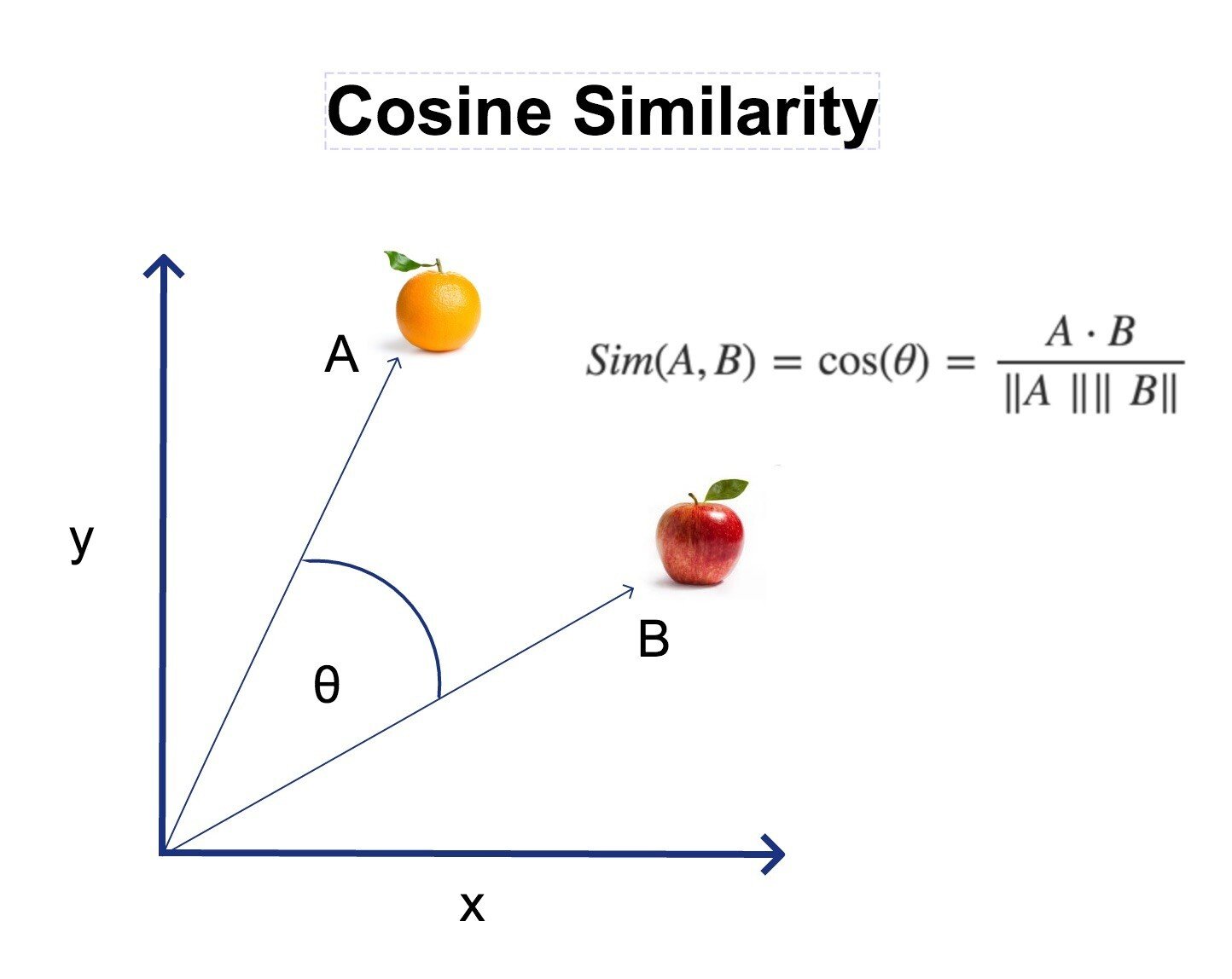
The angle is calculated using cosine similarity , where 1 = 100% similarity (parallell vectors) , and 0 = 0% similarity (perpendicular vectors).
Negative similarity is also possible.
How can I use it?
If you are bored of prompting “girl” and want something similiar you can run this notebook and use the “chick” token at 21.88% similarity , for example
You can also run a mixed search , like “cute+girl”/2 , where for example “kpop” has a 16.71% similarity
There are some strange tokens further down the list you go. Example: tokens similiar to the token "pewdiepie" (yes this is an actual token that exists in CLIP)
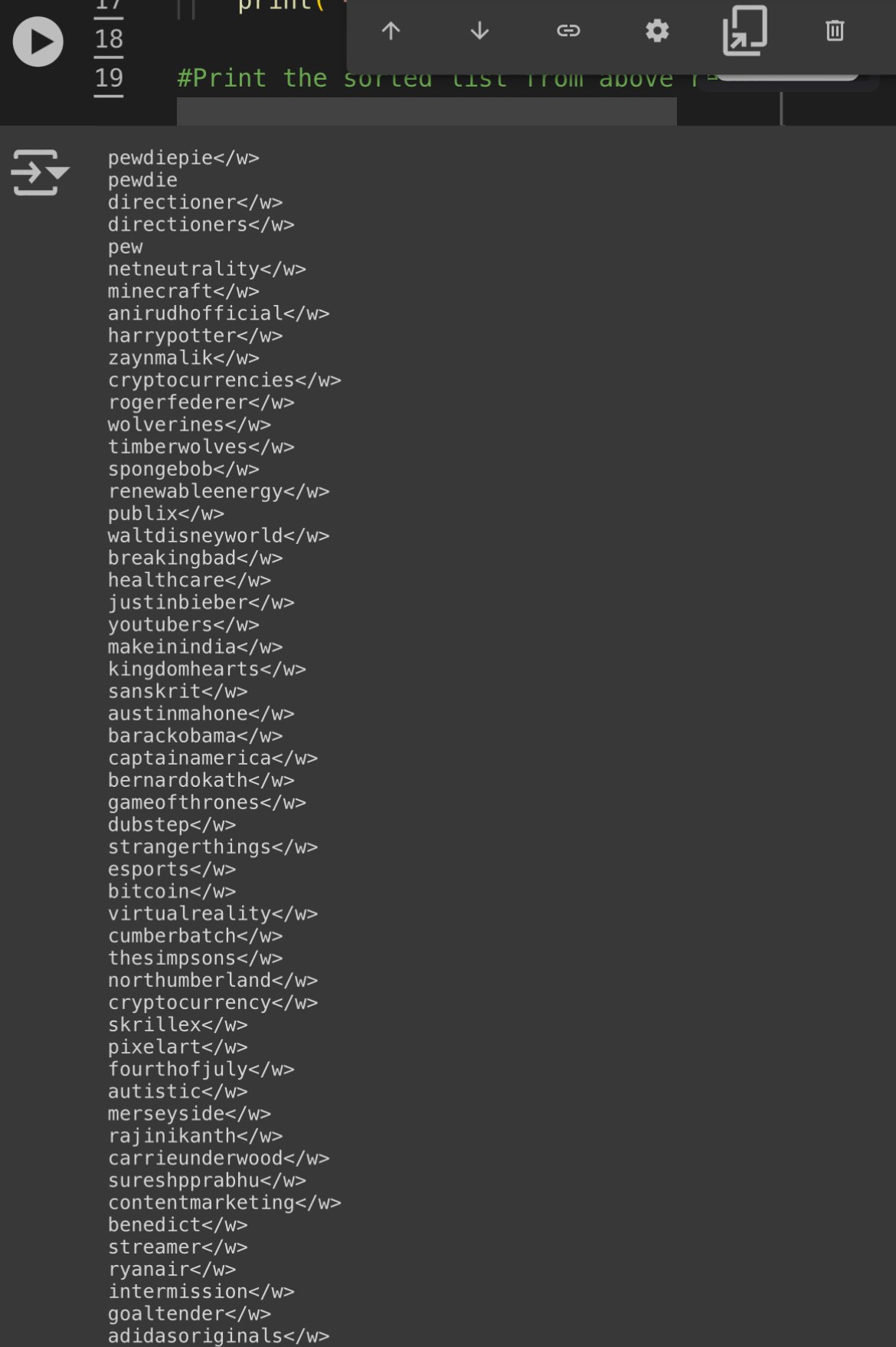
Each of these correspond to a unique 1x768 token vector.
The higher the ID value , the less often the token appeared in the CLIP training data.
To reiterate; this is the CLIP model training data , not the SD-model training data.
So for certain models , tokens with high ID can give very consistent results , if the SD model is trained to handle them.
Example of this can be anime models , where japanese artist names can affect the output greatly.
Tokens with high ID will often give the "fun" output when used in very short prompts.
What about token vector length?
If you are wondering about token magnitude,
Prompt weights like (banana:1.2) will scale the magnitude of the corresponding 1x768 tensor(s) by 1.2 . So thats how prompt token magnitude works.
Source: https://huggingface.co/docs/diffusers/main/en/using-diffusers/weighted_prompts*
So TLDR; vector direction = “what to generate” , vector magnitude = “prompt weights”
How prompting works (technical summary)
-
There is no correct way to prompt.
-
Stable diffusion reads your prompt left to right, one token at a time, finding association from the previous token to the current token and to the image generated thus far (Cross Attention Rule)
-
Stable Diffusion is an optimization problem that seeks to maximize similarity to prompt and minimize similarity to negatives (Optimization Rule)
Reference material (covers entire SD , so not good source material really, but the info is there) : https://youtu.be/sFztPP9qPRc?si=ge2Ty7wnpPGmB0gi
The SD pipeline
For every step (20 in total by default) for SD1.5 :
- Prompt text => (tokenizer)
- => Nx768 token vectors =>(CLIP model) =>
- 1x768 encoding => ( the SD model / Unet ) =>
- => Desired image per Rule 3 => ( sampler)
- => Paint a section of the image => (image)
Disclaimer /Trivia
This notebook should be seen as a "dictionary search tool" for the vocab.json , which is the same for SD1.5 , SDXL and FLUX. Feel free to verify this by checking the 'tokenizer' folder under each model.
vocab.json in the FLUX model , for example (1 of 2 copies) : https://huggingface.co/black-forest-labs/FLUX.1-dev/tree/main/tokenizer
I'm using Clip-vit-large-patch14 , which is used in SD 1.5 , and is one among the two tokenizers for SDXL and FLUX : https://huggingface.co/openai/clip-vit-large-patch14/blob/main/README.md
This set of tokens has dimension 1x768.
SDXL and FLUX uses an additional set of tokens of dimension 1x1024.
These are not included in this notebook. Feel free to include them yourselves (I would appreciate that).
To do so, you will have to download a FLUX and/or SDXL model
, and copy the 49407x1024 tensor list that is stored within the model and then save it as a .pt file.
//---//
I am aware it is actually the 1x768 text_encoding being processed into an image for the SD models + FLUX.
As such , I've included text_encoding comparison at the bottom of the Notebook.
I am also aware thar SDXL and FLUX uses additional encodings , which are not included in this notebook.
//---//
If you want them , feel free to include them yourself and share the results (cuz I probably won't) :)!
That being said , being an encoding , I reckon the CLIP Nx768 => 1x768 should be "linear" (or whatever one might call it)
So exchange a few tokens in the Nx768 for something similiar , and the resulting 1x768 ought to be kinda similar to 1x768 we had earlier. Hopefully.
I feel its important to mention this , in case some wonder why the token-token similarity don't match the text-encoding to text-encoding similarity.
Note regarding CLIP text encoding vs. token
To make this disclaimer clear; Token-to-token similarity is not the same as text_encoding similarity.
I have to say this , since it will otherwise get (even more) confusing , as both the individual tokens , and the text_encoding have dimensions 1x768.
They are separate things. Separate results. etc.
As such , you will not get anything useful if you start comparing similarity between a token , and a text-encoding. So don't do that :)!
What about the CLIP image encoding?
The CLIP model can also do an image_encoding of an image, where the output will be a 1x768 tensor. These can be compared with the text_encoding.
Comparing CLIP image_encoding with the CLIP text_encoding for a bunch of random prompts until you find the "highest similarity" , is a method used in the CLIP interrogator : https://huggingface.co/spaces/pharmapsychotic/CLIP-Interrogator
List of random prompts for CLIP interrogator can be found here, for reference : https://github.com/pharmapsychotic/clip-interrogator/tree/main/clip_interrogator/data
The CLIP image_encoding is not included in this Notebook.
If you spot errors / ideas for improvememts; feel free to fix the code in your own notebook and post the results.
I'd appreciate that over people saying "your math is wrong you n00b!" with no constructive feedback.
//---//
Regarding output
What are the symbols?
The whitespace symbol indicate if the tokenized item ends with whitespace ( the suffix "banana" => "banana " ) or not (the prefix "post" in "post-apocalyptic ")
For ease of reference , I call them prefix-tokens and suffix-tokens.
Sidenote:
Prefix tokens have the unique property in that they "mutate" suffix tokens
Example: "photo of a #prefix#-banana"
where #prefix# is a randomly selected prefix-token from the vocab.json
The hyphen "-" exists to guarantee the tokenized text splits into the written #prefix# and #suffix# token respectively. The "-" hypen symbol can be replaced by any other special character of your choosing.
Capital letters work too , e.g "photo of a #prefix#Abanana" since the capital letters A-Z are only listed once in the entire vocab.json.
You can also choose to omit any separator and just rawdog it with the prompt "photo of a #prefix#banana" , however know that this may , on occasion , be tokenized as completely different tokens of lower ID:s.
Curiously , common NSFW terms found online have in the CLIP model have been purposefully fragmented into separate #prefix# and #suffix# counterparts in the vocab.json. Likely for PR-reasons.
You can verify the results using this online tokenizer: https://sd-tokenizer.rocker.boo/
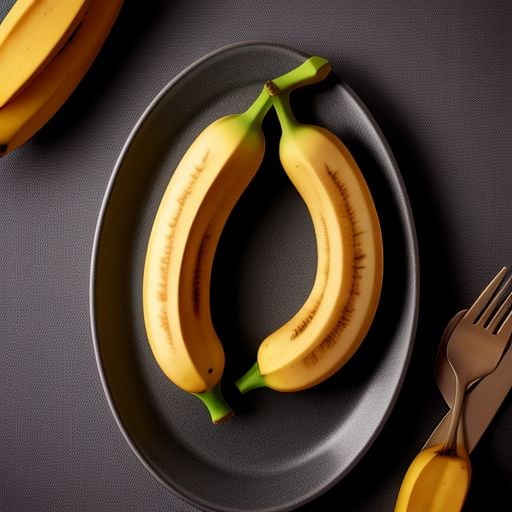
What is that gibberish tokens that show up?
The gibberish tokens like "ðŁĺħ</w>" are actually emojis!
Try writing some emojis in this online tokenizer to see the results: https://sd-tokenizer.rocker.boo/
It is a bit borked as it can't process capital letters properly.
Also note that this is not reversible.
If tokenization "😅" => ðŁĺħ
Then you can't prompt "ðŁĺħ" and expect to get the same result as the tokenized original emoji , "😅".
SD 1.5 models actually have training for Emojis.
But you have to set CLIP skip to 1 for this to work is intended.
For example, this is the result from "photo of a 🧔🏻♂️"
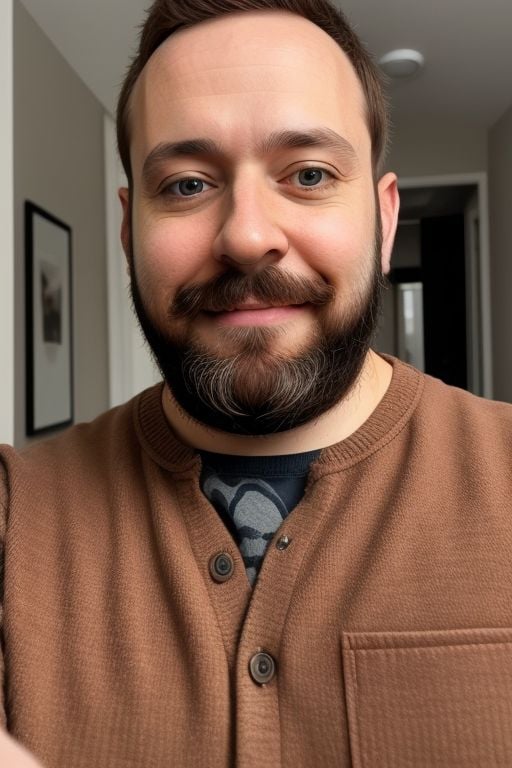
A tutorial on stuff you can do with the vocab.list concluded.
Anyways, have fun with the notebook.
There might be some updates in the future with features not mentioned here.
//---//
My solution has been to left click , select "inspect element" to open the browsers HTML window.
Then zoom out the generator as far as it goes , and scroll down so the entire image gallery (or a part of it at least) is rendered within the browser.
The ctrl+c copy the HTML and paste it in notepad++ , and use regular expressions to sort out the image prompts (and image source links) from the HTML code
Not exactly a good fix , but it gets the job done at least.